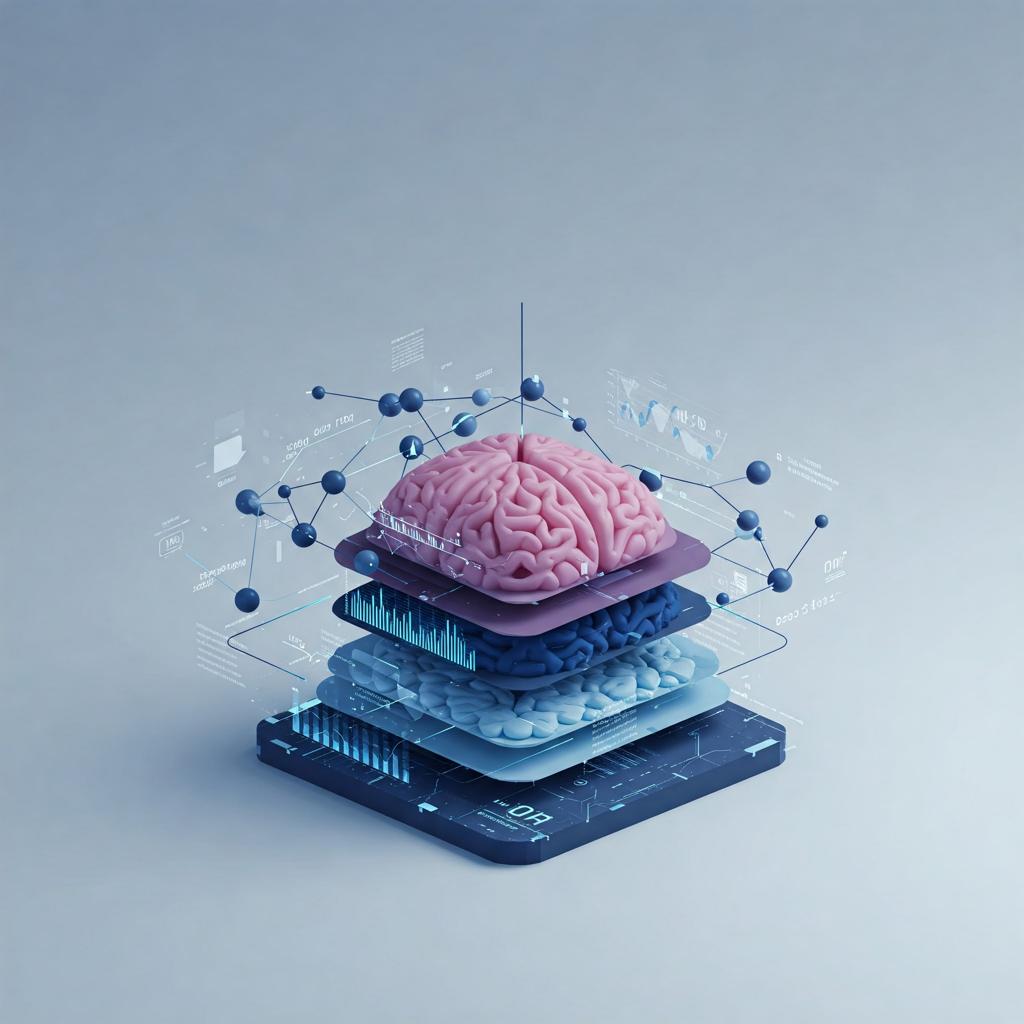
Deep Learning: Revolutionizing AI Algorithms
Deep learning is a subset of machine learning that specializes in leveraging the neural networks with many layers in order to analyze varied data. While typical machine learning models may have only a handful of layers, deep learning algorithms are made up of many layers of connected neurons that mimic the operation of the human brain. These neural networks are capable of learning from extremely large volumes of data and can use that to make predictions, identify patterns, and become optimized over time.
Basic Ideas in Deep Learning
In order to enjoy deep learning, one has to know a few basic principles on which this technology relies. Deep learning is built around neural networks, a computational model reminiscent of the brain. Neural networks consist of layers of nodes, or neurons, in which each node processes a bit of data and forwards it to the next layer.
Deep learning networks are defined by the fact that they have deep architecture, i.e., they have more than one layer between input and output. These layers are usually categorized into an input layer, where raw data feeds into the model, hidden layers that come between input and output and map the data by detecting patterns, and an output layer, the final layer that gives predictions or classifications from the mapped data.
Activation functions are functions that allow us to determine whether a neuron must be activated or not, i.e., whether it should propagate data. Some of the most common activation functions are ReLU (Rectified Linear Unit) and Sigmoid.
Backpropagation is an important process in deep learning where the model adjusts its weights after each prediction to reduce errors. This ensures that the model improves with each iteration and leads to improved predictions.
Deep Learning Model Types
Multiple types of deep learning models are used for a wide range of tasks depending on the nature of the problem to be solved.
Convolutional Neural Networks (CNNs) have direct applications in image and video recognition issues. CNNs are well suited to examine visual data automatically identifying patterns such as edges, texture and shapes at different levels of abstraction. CNNs have extensive applications in scenarios that include face recognition, object identification and medical image processing.
Recurrent Neural Networks (RNNs) are designed to handle sequential data, so the optimal application for tasks such as time series forecasting, speech recognition, and natural language processing (NLP). RNNs consist of a feedback mechanism that allows them to remember previous inputs, which is important in applications that involve sequences, such as speech-to-text solutions or translation.
Generative Adversarial Networks (GANs) are utilized for the generation of new artificial data that closely resembles real data. GANs consist of two neural networks, a generator and a discriminator that collaborate to map input data to images, videos or music. GANs are used widely in scenarios such as image generation, art production and deepfake technology.
Autoencoders are used in unsupervised learning activities, mainly data compression and feature extraction. They work by compressing the input data in a smaller format and then expand it to create the original input. Autoencoders are useful for anomaly detection and dimensionality reduction of big datasets.
Applications of Deep Learning
Deep learning has multiple uses across various industries, transforming the way operations are carried out. In medicine, deep learning is transforming diagnostics, medical imaging and drug discovery. Algorithms powered by deep learning can be applied to scan medical scans such as X-rays and MRIs to identify diseases such as cancer or anomalies.
Deep learning models are also used in genomics for genomic data analysis and identification of new potential treatments.
Deep learning plays a significant role in developing self-driving cars. Through deep models of learning, self-driving cars can learn from real-time camera and sensor data and interpret the data accordingly to recognize objects, pedestrians, road signs and determine how to drive.
Deep models of learning are significant in ensuring the safety and efficiency of self-driving cars. Deep learning has advanced significantly in the field of natural language processing (NLP), enabling machines to understand, decipher, and create human language. Deep learning has been used in many domains of NLP, including language translation, chatbots, voice recognition, and sentiment analysis.
Google's BERT model, for example, uses deep learning for improved language understanding.
In the banking sector, deep learning is used for operations such as fraud detection, stock market movement forecasting and credit rating. Deep learning algorithms are capable of identifying trends in huge quantities of historic financial data that the human eye would overlook, allowing banks to make better decisions and increase security.
Deep learning is also revolutionizing the entertainment industry.
Recommendation systems, for instance, at Netflix and Spotify, rely on deep learning to predict user preference from what they have done before. Deep learning also has applications in video editing, speech synthesis and even game development, to create realistic virtual worlds.
Advantages of Deep Learning
Deep learning enjoys the greatest advantage of extracting features from unprocessed data automatically, without the need to hand engineer features. This makes deep learning highly effective when dealing with intricate high-dimensional data.
Deep learning models are generally more accurate than machine learning models, especially when dealing with tasks such as image classification, speech recognition, and natural language comprehension.
With additional information, deep learning models can further enhance their performance. Deep learning is especially well-suited for handling unstructured information like images, audio, and text. Deep learning models can process large sets of data, enabling more in-depth analysis and better prediction. Deep learning models are highly adaptive and can be customized to meet the specific needs of different industries and functions.
As new data gets incorporated, deep learning models can be retrained so that they are generalizing and more accurate.
Drawbacks of Deep Learning
Despite having numerous advantages, there are tremendous challenges to deep learning. Deep learning models require a lot of labeled data and plenty of computational power in order to be trained. This could be restrictive for organizations that lack adequate resources or good data.
Deep models of learning, particularly deep neural networks, are usually referred to as "black boxes" as it's hard to see how they come to their decisions. Such lack of transparency may be undesirable in areas where explainability is key, like healthcare or finance.
Deep learning models tend to overfit very quickly, especially in cases where there is limited data or where the model is very complex. Regularization techniques have to be employed to ensure that the model will generalize effectively for new data.
The uses of deep learning in facial identification and surveillance have sparked some ethical concerns, among them privacy and misuse. Deep learning technologies have to be applied responsibly.
The Future of Deep Learning
The future of deep learning appears to be rosy, with advancements in hardware, algorithms, and data collection enabling increased improvement in the technology. Scientists keep inventing more effective models that consume less data and computation, and as such, deep learning is becoming increasingly accessible and implementable in an ever-increasing number of industries.
With deep learning increasing, it will also lead to more innovative applications in AI and machine learning.
Deep learning represents a major advance in artificial intelligence technology. Its ability to mimic the sophistication of the human brain and learn from massive data sets has opened up new potential across a wide range of industries, from medicine to entertainment. While there are challenges, the future for deep learning is gigantic with the potential to revolutionize the way we interact with technology and the world in general.