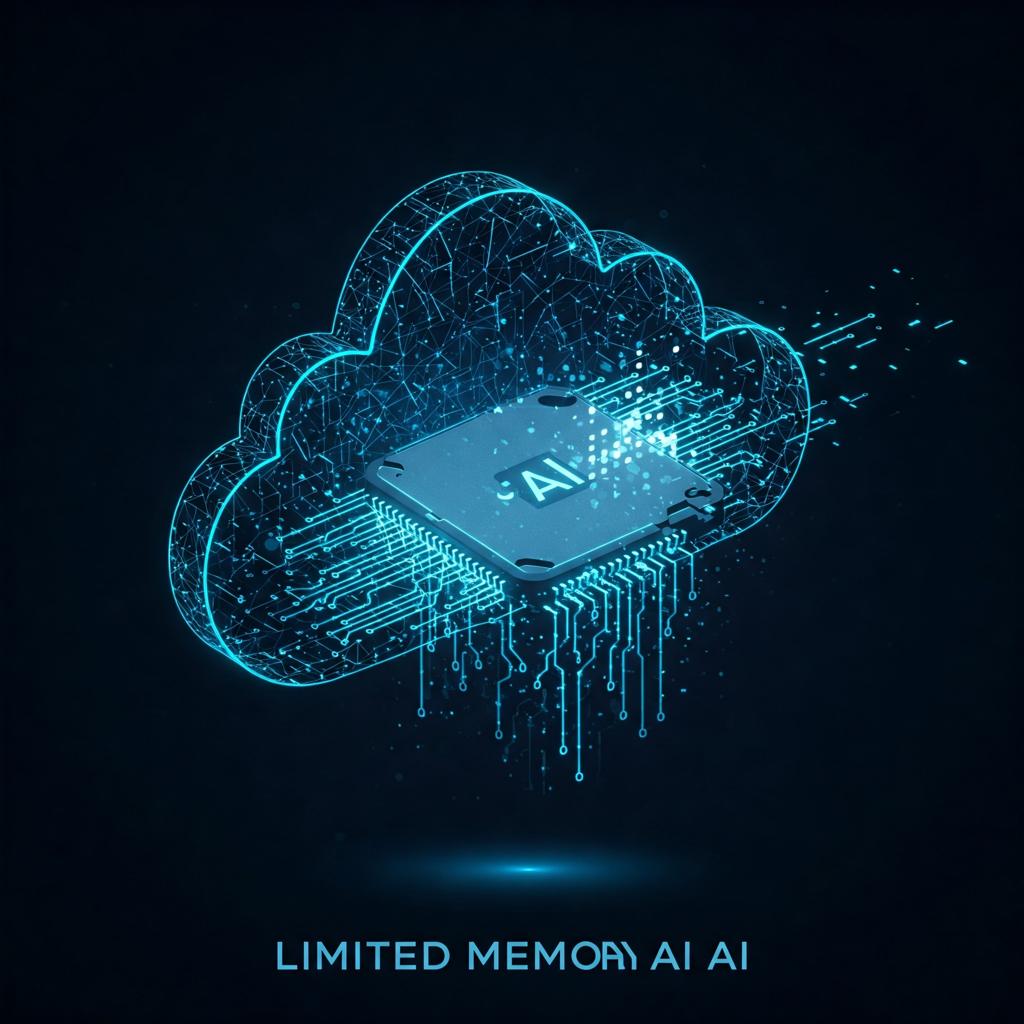
Limited Memory AI: Key Features and Real-World Uses
Artificial intelligence represents a rapidly advancing field, encompassing computational systems designed to perform tasks typically requiring human intellect. Within the diverse landscape of AI, a crucial classification distinguishes systems based on their capabilities, particularly their capacity to utilize past experiences.
Standing significantly beyond purely reactive systems, Limited Memory AI constitutes a class of artificial intelligence capable of retaining information from previous events or data points for a short duration. This retained information directly influences the system's immediate subsequent decisions or actions.
Unlike systems operating solely on current inputs, Limited Memory AI incorporates a temporal dimension, allowing it to perceive sequences and identify patterns unfolding over brief periods. Its functionality hinges on the ability to build upon immediate past observations, creating a more dynamic and contextually aware response mechanism.
This exploration delves into the defining characteristics of Limited Memory AI, examining its operational principles, diverse applications across various sectors, inherent advantages, associated limitations, and its specific position within the broader spectrum of artificial intelligence development.
Understanding this category is fundamental to appreciating the capabilities of many contemporary AI systems that interact with dynamic environments.
Defining the Boundaries: What Constitutes Limited Memory?
The concept of "memory" within this AI classification requires careful delineation. It does not equate to the complex, long-term, associative memory observed in humans. Instead, it refers to the temporary storage and accessibility of specific pieces of information derived from recent inputs or interactions. This stored data persists only for a limited timeframe, directly relevant to the task at hand. The system accesses this transient information cache to inform its processing of new, incoming data, thereby enabling decisions that reflect an awareness of the immediate preceding context.
A stark contrast exists with Reactive Machines, the most basic AI type. Reactive systems function exclusively based on the data presented at the exact moment of decision-making. They possess no mechanism to store or recall past inputs. Think of a simple thermostat reacting only to the current room temperature reading or an early chess program evaluating only the present board state. Limited Memory AI transcends this limitation by incorporating a mechanism, however brief, to look back. This "look back" capability is not comprehensive; it's specifically engineered for task relevance.
The "limited" aspect is paramount. The memory is intentionally constrained in duration and scope. It doesn't build a persistent, accumulating knowledge base about the world in the way a human learns over a lifetime. Instead, it holds onto data points like the recent speed and direction of a nearby vehicle, the last few utterances in a conversation, or the sequence of clicks on a webpage during a single session. The duration might span milliseconds, seconds, minutes, or relate to the completion of a specific event sequence, but it is inherently finite and often overwritten as new data arrives and older data loses its immediate relevance. This temporal restriction prevents the system from drawing upon distant past experiences or developing nuanced, long-term understandings. It operates within a continuously updating, short-term window of observation.
The Inner Workings: How Limited Memory AI Functions
The operational flow of a Limited Memory AI system generally involves several interconnected stages. It begins with data input, typically gathered through sensors or digital interfaces, representing the current state of the environment or interaction. This raw input undergoes initial sensory processing to extract relevant features. Crucially, selected information from this processing, along with information retained from immediately preceding cycles, is held in a temporary data storage component – the system's limited memory.
The core decision-making process then occurs during contextual analysis. Here, new, currently processed input is evaluated in conjunction with the relevant information held in the temporary storage. This allows the system to interpret the present situation not in isolation, but as part of an unfolding sequence. For instance, observing a car ahead is one thing; observing it while recalling its immediately preceding deceleration provides crucial context for predicting its likely future action.
This contextual analysis often involves comparing current data against patterns learned from vast datasets during a prior training phase, but the application of these learned patterns is modulated by the specific recent history stored in memory. The output of this analysis is a decision or an action, which could range from adjusting steering angle in a vehicle, suggesting a related product online, formulating a relevant response in a chat, or flagging a potential anomaly in sensor readings.
An essential aspect of this process is the continuous updating of the system's transient "world model" or operational context. As new information arrives and actions are taken, the limited memory cache is refreshed. Older, less relevant data points might be discarded (or fade in influence) to make way for newer observations. This ensures the system primarily bases its actions on the most immediate and pertinent past events, aligning with the "limited" nature of its memory. While the system relies on pre-programmed rules and patterns learned offline, its real-time performance is significantly shaped by this dynamic, short-term memory mechanism that allows it to adapt to sequential nuances. The algorithms enabling this often involve architectures specifically designed for processing data where order and time are critical factors.
Real-World Manifestations: Applications Across Industries
The capability to leverage recent past information makes Limited Memory AI exceptionally valuable in domains where conditions change dynamically and context is key. Its applications are widespread, underpinning many sophisticated systems we interact with daily.
- Autonomous Vehicles: This is perhaps the most cited example. Self-driving cars continuously process data from cameras, LiDAR, radar, and other sensors. Their Limited Memory capabilities are essential for safe navigation. The system doesn't just see a car ahead; it remembers its speed and trajectory over the last few seconds to anticipate whether it might change lanes. It tracks the positions and movements of multiple pedestrians and cyclists nearby, using their recent paths to predict potential crossings. Observing the sequence of traffic light changes (green to yellow) informs the decision to proceed or brake. Lane keeping relies on continuously comparing the vehicle's current position relative to lane markings observed fractions of a second earlier. This short-term recall is fundamental for interpreting complex traffic scenarios and making split-second, safety-critical decisions based on evolving dynamics rather than static snapshots.
- Recommendation Systems: Modern e-commerce sites and streaming platforms heavily utilize Limited Memory AI to personalize user experiences in real-time. Beyond your general profile, these systems track your clicks, viewed items, added-to-cart products, or watched videos during the current session. If you browse several sci-fi movies, the system's limited memory notes this recent trend and starts prioritizing suggestions within that genre immediately, adapting its recommendations far quicker than if it only relied on your long-term viewing history. This short-term interaction history provides dynamic context that significantly refines the relevance of presented options.
- Chatbots and Virtual Assistants: Engaging in a coherent conversation requires remembering what was just said. Advanced chatbots employ Limited Memory to maintain the context of the ongoing dialogue. They recall the user's previous questions or statements within the same session to provide relevant answers, ask clarifying questions, or complete multi-step tasks. A bot assisting with a booking needs to remember the destination and dates mentioned earlier in the conversation to process the final request. This ability to track the conversational thread distinguishes them from simple reactive bots that treat each query as an isolated event, resulting in more natural and less frustrating user interactions.
- Financial Modeling and Algorithmic Trading: Financial markets are inherently sequential and time-sensitive. AI systems used for market analysis or automated trading rely on Limited Memory to process recent price fluctuations, trading volumes, news releases, and other relevant data points occurring over minutes, hours, or days. Identifying short-term trends, momentum shifts, or arbitrage opportunities often depends on analyzing the immediate sequence of events. The system's memory allows it to factor in the very recent market behaviour when making predictions or triggering buy/sell orders.
- Healthcare Monitoring: In clinical settings, AI systems can monitor patient vital signs (heart rate, blood pressure, oxygen saturation) continuously. Limited Memory allows these systems to analyze trends over short periods. A single abnormal reading might be insignificant, but a pattern of readings showing a consistent upward or downward trend over the last hour, held within the system's memory, could indicate a developing issue requiring medical attention. It detects patterns in the temporal sequence of physiological data.
- Industrial Automation and Robotics: Robots operating in manufacturing or logistics often need to adapt to slight variations in their environment or tasks. A robot on an assembly line might use sensors to detect the precise orientation of a part it needs to pick up. Its limited memory could store information about the previous few parts handled, potentially allowing it to adjust its grasp or approach based on recent minor variations or feedback from downstream quality checks. This short-term adaptation enhances efficiency and reduces errors in dynamic production environments.
- Video Game AI: Non-player characters (NPCs) in video games can appear more intelligent and responsive through Limited Memory. An enemy NPC might remember the player's last known location for a short period, allowing it to conduct a more believable search pattern. An ally NPC might react based on the player's recent actions, such as healing them or attacking the same target. This short-term memory makes the game world feel more interactive and less predictably scripted.
These examples illustrate how the core capability of referencing the immediate past enables AI systems to operate more effectively, safely, and intuitively in complex, time-dependent scenarios across a multitude of fields.
Strengths and Advantages: The Value Proposition
The incorporation of even a limited temporal scope provides significant advantages over purely reactive approaches, making Limited Memory AI a powerful tool.
- Improved Decision-Making: By considering the immediate context provided by recent past events, these systems can make more informed, relevant, and nuanced decisions. They are not confined to reacting solely to the present stimulus but can interpret it within the framework of what just happened. This leads to actions that are often more appropriate and effective in sequential tasks.
- Enhanced Performance in Dynamic Environments: The real world is rarely static. Limited Memory AI excels in situations where conditions change over time. Its ability to track recent changes allows it to adapt its behaviour accordingly, whether navigating shifting traffic patterns, responding to evolving user needs in a conversation, or adjusting to fluctuations in financial markets.
- More Natural Interactions: For systems designed to interact with humans, such as chatbots or virtual assistants, maintaining a short-term memory of the conversation flow is crucial. It prevents repetitive questioning and allows the system to build upon previous exchanges within the same session, leading to interactions that feel smoother, more coherent, and less robotic.
- Effective Handling of Sequential Data: Many important real-world tasks involve data where the order of information is critical (e.g., language, time series data, video). Limited Memory AI architectures are specifically designed to process such sequences, capturing temporal dependencies that would be lost if each data point were treated in isolation.
- Foundation for More Complex AI: While limited in its scope, this type of memory represents a fundamental step beyond reactive processing. It serves as a necessary building block and conceptual stepping stone towards developing more advanced AI systems that might incorporate longer-term memory or more sophisticated reasoning capabilities. Mastering the handling of short-term temporal context is vital for future progress.
- Increased Task Complexity Handling: Tasks that inherently involve sequences or require tracking changes over time (e.g., object tracking, gesture recognition, process monitoring) become feasible and perform significantly better with Limited Memory capabilities compared to reactive systems.
These strengths highlight why Limited Memory AI is currently the state-of-the-art for a vast array of practical AI applications, bridging the gap between simple stimulus-response mechanisms and the more complex cognitive functions associated with higher forms of intelligence.
Constraints and Challenges: Understanding the Limitations
Despite its advantages over reactive systems, Limited Memory AI possesses inherent constraints and faces several challenges that define the boundaries of its capabilities.
- Limited Temporal Scope: The most defining limitation is in the name itself. The memory is transient and typically restricted to a relatively short, task-specific timeframe. These systems cannot access or utilize information from the distant past. They don't build cumulative knowledge or learn from experiences outside their predefined memory window. This prevents them from understanding long-term cause-and-effect, developing deep historical context, or engaging in planning that requires recalling distant events.
- Data Dependency and Quality: The performance of Limited Memory AI is highly contingent on the continuous stream of recent data it receives. If the input data is noisy, incomplete, or biased, the system's short-term memory will reflect these flaws, potentially leading to poor decisions. Furthermore, the system primarily learns patterns from the data it's trained on and the recent data it processes; it doesn't possess general world knowledge to compensate for data gaps or anomalies.
- Model Complexity and Training: Implementing and training AI models capable of effectively managing even limited memory, such as certain types of neural networks, can be computationally expensive and require significant amounts of labeled sequential data. Tuning these models to optimally balance memory retention and forgetting is often a complex engineering challenge.
- Risk of Outdated Information: In highly volatile environments where critical changes occur faster than the system's memory refresh cycle or outside the scope of its attention, the system might base its decisions on recent but already obsolete information. The "memory" might lag slightly behind reality, which can be problematic in safety-critical applications.
- Lack of True Understanding or Consciousness: It is crucial to recognize that despite using past information, Limited Memory AI does not possess genuine comprehension, consciousness, or subjective experience. Its processing is based on sophisticated pattern matching on sequential data, not on understanding the meaning behind the information or forming beliefs about the world. It simulates contextual awareness within a narrow scope.
- Scalability Issues: As the required memory window duration increases or the complexity of the sequential patterns grows, managing and processing the necessary state information can become computationally demanding, potentially limiting the scalability of certain approaches for very long sequences or extremely high-dimensional data streams.
- Difficulty with Generalization to Novel Sequences: While trained to recognize patterns in sequences, these systems can struggle when faced with entirely novel types of sequences or abrupt shifts in underlying dynamics that were not represented in their training data or recent history. Their adaptability is powerful but constrained by experience.
Understanding these limitations is essential for deploying Limited Memory AI systems appropriately, managing expectations about their capabilities, and guiding research towards overcoming these challenges in the pursuit of more advanced artificial intelligence.
Enabling Technologies and Algorithms
The ability of Limited Memory AI to retain and utilize recent information is not magic; it is enabled by specific computational architectures and algorithms designed to handle sequential data.
The process typically starts with Sensors (in physical systems like robots or cars) or data interfaces (in software) that gather the raw input stream representing the environment or interaction over time. The quality and type of sensors directly impact the richness of the data available for memory formation.
At the core of processing sequential information lie specialized neural network architectures:
- Recurrent Neural Networks (RNNs): These are foundational networks designed with loops, allowing information to persist from one step (or time point) in the sequence to the next. In essence, the output from processing one element in a sequence is fed back as an input when processing the next element. This creates a basic form of memory, where the network's current state is influenced by its immediately preceding states. Imagine reading a sentence: an RNN processes each word while retaining some "memory" of the words that came before it, helping it understand the context. However, basic RNNs struggle to maintain relevant information over longer sequences due to issues like the vanishing gradient problem, where the influence of earlier inputs fades too quickly.
- Long Short-Term Memory (LSTM) Units: LSTMs are a sophisticated type of RNN specifically designed to overcome the short-term memory limitations of basic RNNs. They incorporate internal mechanisms called "gates" (input gate, forget gate, output gate). These gates act like regulators, controlling the flow of information. The forget gate decides which information from the previous state should be discarded, the input gate determines what new information should be added, and the output gate controls what part of the current state is outputted. This gating structure allows LSTMs to selectively remember relevant information for much longer periods and forget irrelevant details, making them highly effective for tasks involving complex, long-range temporal dependencies, such as machine translation or speech recognition.
- Gated Recurrent Units (GRUs): GRUs are a variation on LSTMs, also using gating mechanisms but with a slightly simpler architecture (typically combining the forget and input gates into an "update gate" and using a "reset gate"). They offer similar performance to LSTMs on many tasks but can be computationally slightly more efficient due to having fewer parameters. Like LSTMs, they enable the network to learn which information to retain and which to discard over time, forming the basis of the system's limited memory.
While RNNs, LSTMs, and GRUs are central, other techniques also contribute. Attention Mechanisms, often used in conjunction with sequence models (like in Transformer architectures, though Transformers themselves don't inherently have recurrence in the same way), allow the model to dynamically focus on specific parts of the input sequence (or the system's memory) that are most relevant for making the current decision, effectively assigning different weights to past information. Certain Reinforcement Learning approaches also incorporate short-term memory by including recent observations or actions as part of the "state" representation used by the learning agent to make decisions.
These technologies provide the computational toolkit necessary to build systems that don't just react to the present but incorporate the immediate past into their operational logic.
Positioning Limited Memory AI in the AI Spectrum
Understanding Limited Memory AI requires placing it within the widely discussed framework of AI classification based on capability, often categorized into four types:
- Type I: Reactive Machines: These are the simplest AI systems. As previously discussed, they operate solely based on current input, lacking any form of memory or ability to use past experiences. Examples include IBM's Deep Blue chess computer (evaluating the current board state) or basic spam filters looking at individual email content without context from previous emails. Limited Memory AI represents a significant advancement beyond this type by introducing the crucial element of short-term data retention.
- Type II: Limited Memory: This is the category currently under discussion. These systems can store and use past information, but only for a short duration and typically for a specific task. Most of the sophisticated AI applications currently in use fall into this category, from autonomous vehicles navigating traffic to virtual assistants recalling the last sentence you spoke. They demonstrate improved performance in dynamic environments compared to reactive machines but lack broader context or understanding.
- Type III: Theory of Mind (Hypothetical): This represents a future, more advanced stage of AI. Theory of Mind AI would possess the ability to understand and attribute mental states—beliefs, intentions, desires, emotions, and knowledge—to themselves and others. They could comprehend that other entities have their own thoughts and feelings that influence their behaviour. Current AI, including Limited Memory systems, lacks this capability entirely. Limited Memory AI processes past actions or data points but does not infer the underlying beliefs or intentions driving those actions in others. Achieving Theory of Mind remains a major research challenge.
- Type IV: Self-Awareness (Hypothetical): This is the pinnacle of the classification, denoting AI systems that have consciousness, sentience, and a sense of self. A self-aware AI would understand its own existence, internal states, and potentially possess subjective experiences. This is currently confined to the realm of science fiction and philosophical debate. Limited Memory AI operates purely on data processing and pattern matching based on recent inputs; it has absolutely no self-concept, consciousness, or subjective awareness.
Therefore, Limited Memory AI occupies a critical position as the most advanced currently realized type of AI in widespread practical application. It bridges the gap between purely reactive systems and the yet-unachieved capabilities of understanding mental states or possessing consciousness. It represents the cutting edge of deployed AI while still being fundamentally limited compared to the complexities of human cognition or the hypothetical future types of AI.
Ethical Considerations and Societal Impact
The deployment of Limited Memory AI systems, while beneficial, raises several important ethical considerations and has significant societal impacts that warrant careful attention.
- Bias Amplification from Recent Data: Since these systems learn from and adapt based on recent data, they can potentially pick up and perpetuate biases present in that data stream. If recent interactions or environmental data reflect societal biases, the AI's short-term memory might lead it to make decisions that reinforce those biases in real-time, potentially leading to unfair or discriminatory outcomes.
- Autonomous Decision-Making in Edge Cases: Systems like autonomous vehicles rely heavily on their limited memory to navigate complex situations. However, their memory is finite and based on past training and recent observations. In unforeseen "edge case" scenarios not adequately covered during training or falling outside the normal patterns of recent data, the decisions made based solely on this limited context could be unpredictable or suboptimal, with potentially serious consequences. How these systems prioritize conflicting information within their memory window in critical moments is a key ethical challenge.
- Transparency and Explainability: Understanding precisely why a Limited Memory AI system made a particular decision can be difficult. While we know it uses recent past information, tracing the exact influence of specific data points within its memory cache on the final output can be complex, especially with intricate models like LSTMs. This lack of complete transparency can be problematic in situations requiring accountability or debugging.
- Dependence and Over-reliance: As these systems become more capable and integrated into daily life (e.g., navigation, recommendations, assistance), there is a risk of over-reliance. Users might implicitly trust the system's context-aware decisions without fully appreciating the limitations of its memory or its inability to grasp broader context or long-term implications.
- Data Privacy Concerns: Systems that maintain even a short-term memory of user interactions (like chatbots or recommendation engines) inherently collect and process personal data. Ensuring this data is handled securely, used ethically, and that users have control over their information, even if it's only stored temporarily, remains a crucial privacy consideration.
- Job Displacement and Skill Shifts: As AI capable of handling dynamic, sequential tasks becomes more prevalent in areas like customer service, logistics, and monitoring, it can automate tasks previously performed by humans, leading to potential job displacement and necessitating workforce adaptation towards skills that complement AI capabilities.
Addressing these ethical considerations requires ongoing research into bias detection and mitigation, robust testing methodologies (especially for edge cases), development of more explainable AI techniques, clear communication about system limitations, strong data governance policies, and proactive strategies for managing the societal transitions accompanying AI adoption.
The Path Forward: Evolution and Future Trends
Limited Memory AI represents a significant milestone, but it is not the endpoint of AI development. Research and innovation continue to push the boundaries, suggesting several potential directions for the evolution of this capability and its role in future AI systems.
- Longer and More Sophisticated Memory: A primary focus is extending the duration and increasing the sophistication of AI memory. While still falling short of human-like long-term memory, future systems might incorporate mechanisms to retain relevant information for longer periods or across different tasks, potentially using hierarchical memory structures or integrating memory modules with different temporal scopes.
- Improved Algorithms: Ongoing research aims to develop more efficient and effective algorithms for managing memory in AI. This includes advancements in LSTM and GRU architectures, novel approaches like Transformer models (which use attention to handle long-range dependencies, sometimes complementing or replacing recurrent memory), and techniques for more efficient memory compression and retrieval.
- Integration with Other AI Techniques: Future systems are likely to involve hybrid approaches, combining the strengths of Limited Memory AI (for handling dynamic sequences) with other AI paradigms. This could include integrating short-term memory with large knowledge graphs (for factual grounding), symbolic reasoning engines (for logical deduction), or more advanced reinforcement learning techniques that build more complex world models over time.
- Enhanced Contextual Understanding: While true Theory of Mind is distant, research explores ways to enable AI to build richer contextual models from their limited memory. This might involve not just remembering what happened recently, but also developing a rudimentary understanding of why it might have happened within the learned patterns of the environment, leading to more robust predictions and decisions.
- Energy Efficiency: Processing sequential data and maintaining memory states can be energy-intensive. A key trend is the development of more energy-efficient hardware (neuromorphic chips) and algorithms specifically designed for low-power execution of memory-augmented AI models, crucial for deployment on edge devices like mobile phones or sensors.
- Towards More General Capabilities: Limited Memory AI provides a crucial capability for dealing with the temporal nature of the real world. Continued progress in this area, alongside advancements in other AI domains like perception, reasoning, and learning, forms part of the broader, long-term quest towards Artificial General Intelligence (AGI) – systems with human-like cognitive abilities across a wide range of tasks. While AGI remains a distant goal, enhancing memory capabilities is seen as a necessary step along that path.
The trajectory suggests that AI systems will become increasingly adept at leveraging past information, moving beyond purely reactive responses and short-term recall towards more integrated and contextually aware forms of intelligence, continually reshaping how machines interact with and operate within our world.