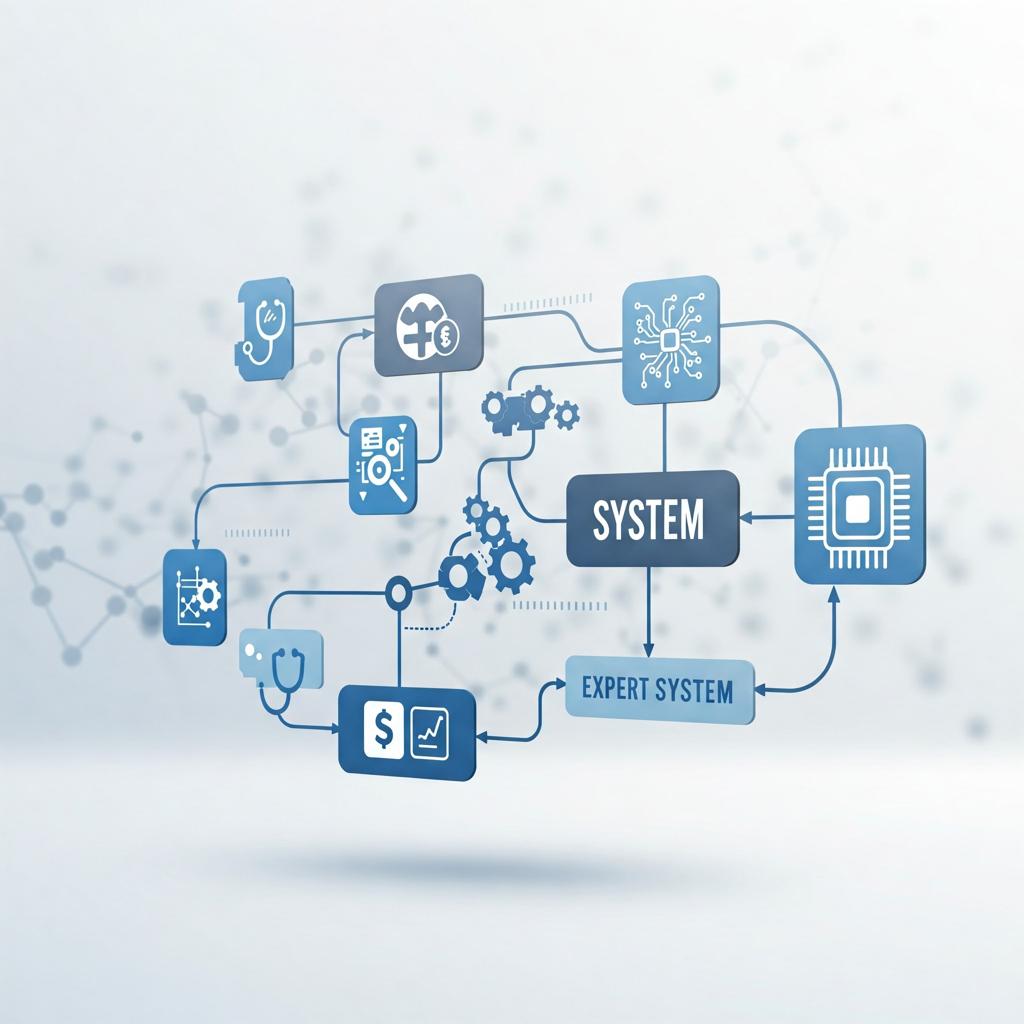
Logic-Based AI: Enhancing Explainability and Trust
Expert systems are a specialized subset of artificial intelligence (AI) that mimic the decision-making abilities of human experts in specific fields. These systems use pre-defined rules and logical frameworks to solve problems or offer solutions in complex domains. Unlike machine learning or neural network models, expert systems are not designed to learn from data over time but instead rely on the knowledge explicitly programmed into them. This makes them ideal for well-defined, structured environments where decision-making can be based on clear rules.
Key Concepts of Expert Systems
The foundation of an expert system lies in several key components that enable it to function effectively. These components define how the system interprets input data and generates decisions or solutions based on that data.
Knowledge Base
At the heart of an expert system is its knowledge base, which contains all the facts and rules necessary for decision-making. The knowledge base consists of a collection of domain-specific information, typically structured in the form of "if-then" rules. For example, in a medical expert system, the knowledge base might include rules that link symptoms to potential diagnoses. The accuracy and completeness of the knowledge base are crucial for the system’s performance, as the system's effectiveness is directly tied to the quality of this knowledge.
Inference Engine
The inference engine acts as the logical engine of the expert system. It is responsible for processing the rules in the knowledge base in conjunction with the input data to generate conclusions. The inference engine applies logical reasoning to determine which rules are relevant and how they should be applied. There are two common types of reasoning mechanisms used in inference engines: forward chaining and backward chaining.
Forward Chaining: This starts with known facts and applies rules to infer new information or conclusions.
Backward Chaining: This begins with a goal or hypothesis and works backward to identify the facts or conditions needed to support the goal.
User Interface
The user interface allows users to interact with the expert system. This could be through inputting data, asking questions, or receiving output. The user interface is often designed to be intuitive and accessible, even for users without specialized knowledge of the domain. It is essential that the user interface is easy to use, as it ensures that non-experts can effectively interact with the system and benefit from its expertise.
Explanation System
One of the distinguishing features of expert systems is their explanation capability. Many expert systems provide an explanation feature that justifies their conclusions or recommendations. This is crucial, particularly in fields where decisions can have significant consequences, such as healthcare or law. An explanation system provides transparency by outlining the reasoning process behind a conclusion, thus helping users trust and understand the output provided by the expert system.
How Expert Systems Work
Expert systems operate through a structured series of steps that combine knowledge from the knowledge base and reasoning from the inference engine to arrive at decisions or solutions. Here is a simplified breakdown of how an expert system typically works:
Input Data
The user provides input data, which might include symptoms, conditions, or other relevant information about a specific problem. This data is processed by the expert system to determine the appropriate action or recommendation.
Rule Application
The system’s inference engine then examines the input data against the rules in the knowledge base. Based on the rules, the system identifies which conditions are met and applies the relevant rules to generate conclusions or solutions.
Inference
Using the rules, the system makes logical deductions to reach a conclusion. It may use forward or backward chaining, depending on the approach used by the system. The inference process ensures that the conclusions drawn are consistent with the rules and the input data.
Conclusion or Solution
Once the inference engine has processed the rules and facts, the expert system generates a conclusion, recommendation, or solution. This could be a diagnosis, a decision, or an action to take based on the available information.
User Feedback
Some expert systems also incorporate feedback loops, where users can refine their input or provide additional information, leading to a more accurate or relevant conclusion. This feedback helps improve the system's overall accuracy and usefulness.
Types of Expert Systems
Expert systems can be categorized into different types based on their reasoning approaches or the way they represent knowledge. The three most common types are rule-based, frame-based, and hybrid expert systems.
Rule-Based Expert Systems
Rule-based expert systems are the most common type and use a series of "if-then" rules to make decisions. These rules are derived from the expertise of human specialists in the given domain. Rule-based expert systems are particularly useful in domains where well-defined, structured rules can be used to solve problems, such as medical diagnosis or technical troubleshooting.
Frame-Based Expert Systems
Frame-based expert systems represent knowledge in structures known as frames. A frame is a data structure that holds information about a particular concept and can be used to describe relationships between objects or entities in the domain. These systems are well-suited for domains that require detailed, hierarchical representations of knowledge, such as engineering design or architectural planning.
Hybrid Expert Systems
Hybrid expert systems combine multiple reasoning techniques to address complex problems. These systems may integrate rule-based reasoning with machine learning, neural networks, or other AI techniques. Hybrid systems are particularly useful when a single method is not sufficient to handle the complexity of a given problem. For example, in robotics, a hybrid system might combine rule-based decision-making with machine learning to adapt to new environments.
Applications of Expert Systems
Expert systems are used in a wide range of fields to provide decision support and solve complex problems. Here are some notable applications of expert systems:
Healthcare and Medical Diagnosis
In healthcare, expert systems are widely used to assist in medical diagnosis and treatment planning. These systems can analyze patient data, symptoms, and medical history to suggest possible diagnoses or treatments. The MYCIN expert system, for instance, was an early example of a system designed to assist doctors in diagnosing bacterial infections and recommending appropriate antibiotics.
Finance and Banking
In finance, expert systems are used for various tasks, including credit scoring, fraud detection, and risk assessment. By analyzing historical financial data, these systems can predict the likelihood of loan repayment, detect fraudulent transactions, and provide financial advice.
Customer Support and Troubleshooting
Expert systems are commonly employed in customer service for troubleshooting technical issues or providing product support. These systems guide users through diagnostic steps and offer solutions based on the information provided by the user. They are widely used in IT support and other technical industries.
Engineering and Design
In engineering, expert systems are used for tasks such as product design, system optimization, and fault detection. These systems can analyze complex engineering data and provide recommendations on design improvements, process optimizations, or maintenance schedules.
Legal Reasoning
In the legal field, expert systems assist in case analysis and legal research. These systems help lawyers navigate complex legal frameworks, statutes, and case law to generate recommendations or strategies for specific legal issues.
Advantages of Expert Systems
Expert systems offer numerous benefits, making them valuable tools in a variety of industries. Some of the key advantages include:
Expert-Level Knowledge
Expert systems enable organizations to access high-level expertise without requiring a human expert for every decision. This can help organizations solve complex problems efficiently and consistently.
Consistency and Accuracy
Since expert systems follow predefined rules, they ensure that decisions are consistent and based on the same criteria each time. This reduces the likelihood of errors caused by human biases or fatigue.
Availability and Efficiency
Expert systems are available 24/7 and can process large volumes of information in a short period. This makes them highly efficient, particularly for tasks that require quick decision-making, such as customer service or fraud detection.
Cost-Effectiveness
By automating decision-making processes, expert systems can help organizations reduce the need for human intervention and, in turn, lower operational costs. This is particularly valuable for businesses with high-volume tasks that require consistent decision-making.
Challenges of Expert Systems
Despite their advantages, expert systems face certain challenges that limit their applicability and effectiveness in some domains.
Limited Knowledge Base
Expert systems are only as good as the knowledge base they rely on. If the rules and facts within the knowledge base are outdated or incomplete, the system may provide inaccurate or suboptimal solutions. Keeping the knowledge base current can be a significant challenge.
Difficulty in Capturing Tacit Knowledge
Tacit knowledge, or knowledge that is difficult to articulate or formalize, is often challenging to encode into an expert system. This can limit the scope of the system's expertise, as certain complex or nuanced insights may be difficult to translate into explicit rules.
Complexity and Maintenance
As expert systems grow in complexity, maintaining and updating their knowledge base becomes increasingly resource-intensive. Keeping the system up to date and ensuring that it remains accurate can require significant time and effort.
Lack of Adaptability
Unlike machine learning systems, expert systems do not have the ability to learn from new data or adapt to changes in the environment. This makes them less flexible than other types of AI systems, particularly in dynamic or rapidly evolving domains.
In conclusion, expert systems represent a powerful tool in artificial intelligence, offering valuable insights and decision support across a wide range of industries. While they have their challenges, their ability to replicate human expertise makes them indispensable for many complex problem-solving tasks.