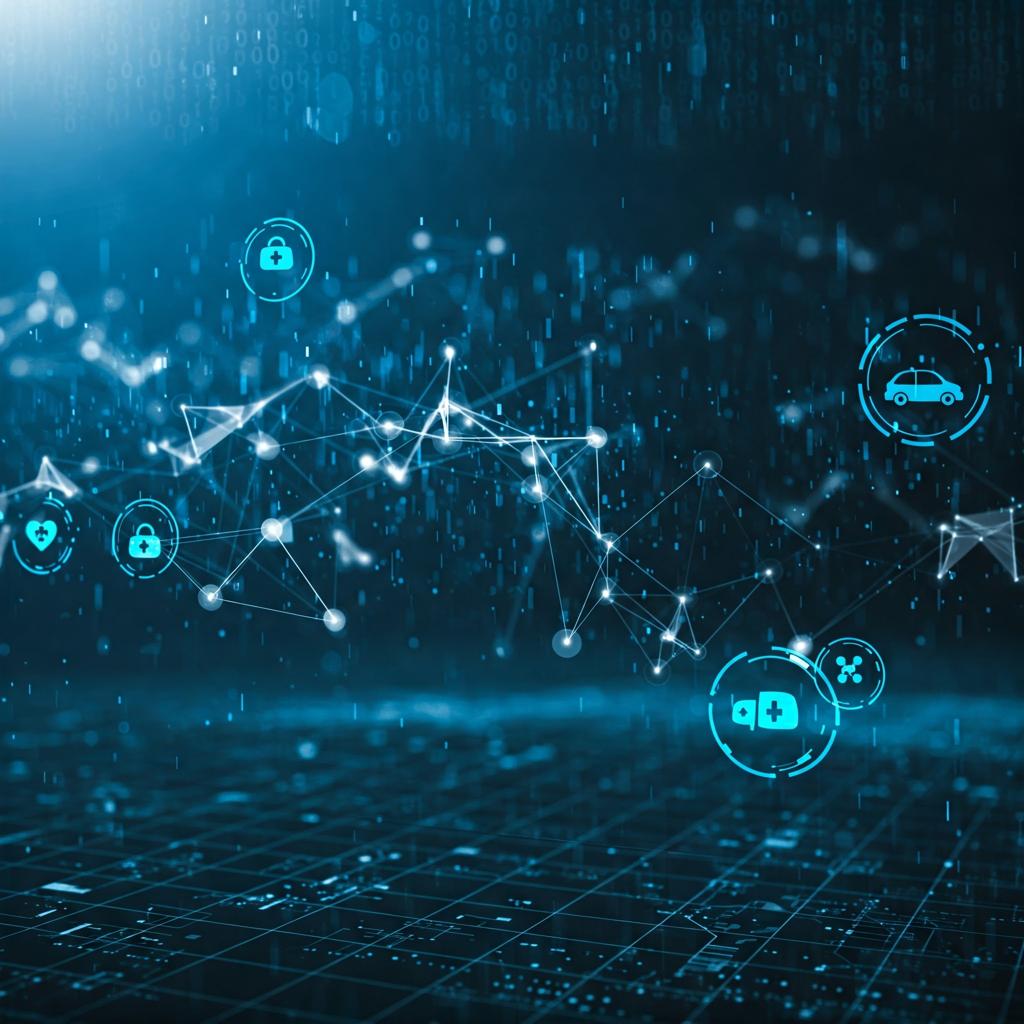
Machine Learning in the Flow of Intelligence
Machine Learning (ML) refers to a specialized area within artificial intelligence (AI) that involves the construction of systems capable of identifying statistical patterns in data and generating responses or actions based on that information. Unlike rule-based systems, ML models operate through algorithmic strategies that evaluate data sets and progressively adjust their internal structures to enhance performance. This computational adaptability enables these systems to deliver outputs without explicit human-designed logic pathways.
Key Concepts of Machine Learning
A foundational understanding of machine learning begins with grasping the components that define its operation. These elements form the basis upon which various algorithms function and evolve.
Training Data
Training data constitutes the initial input fed into a learning algorithm. It includes numerous examples that represent the target task, whether classification, regression, or other data-driven objectives. The variety, quality, and size of training data significantly influence model accuracy and adaptability.
Model
The model in machine learning is the configuration created by an algorithm once it processes training data. This structure maps input variables to output predictions or classifications, serving as the operational core of any ML application.
Algorithm
An algorithm is a formalized method for interpreting data, identifying regularities, and updating the model’s internal state. Different algorithms offer varying levels of efficiency, precision, and suitability for specific types of data.
Features
Features are distinct measurable elements within the dataset that are relevant to the task the model aims to perform. In a dataset used to evaluate housing prices, for example, features may include square footage, neighborhood, and number of rooms.
Labels
Labels refer to the output values associated with training samples in supervised learning scenarios. They represent known outcomes, allowing the model to compare its predictions against actual results during the training phase.
Types of Machine Learning
Machine learning can be categorized into primary paradigms, each defined by the nature of the data provided and the desired outcomes.
Supervised Learning
Supervised learning involves algorithms that are trained using labeled datasets. In this context, each example in the training set includes an input vector and a corresponding output value. The model learns to map inputs to outputs accurately.
Examples of Supervised Algorithms
Linear Regression: Predicts numeric values using input variables.
Logistic Regression: Performs binary classification tasks.
Decision Trees: Splits data based on attribute values to reach conclusions.
Support Vector Machines: Constructs hyperplanes in feature space for classification tasks.
Unsupervised Learning
In unsupervised learning, the system is exposed to input data without predefined labels. The model attempts to uncover inherent structure or groupings within the dataset.
Examples of Unsupervised Algorithms
K-Means Clustering: Partitions data into groups with similar characteristics.
Hierarchical Clustering: Builds nested clusters for hierarchical structure analysis.
Principal Component Analysis (PCA): Reduces dimensionality by identifying essential data features.
Reinforcement Learning
Reinforcement learning involves an agent that operates in an environment and learns to achieve goals through trial and error. Rewards and penalties guide the agent’s decisions and strategy refinement.
Key Reinforcement Learning Concepts
Agent: The decision-making system.
Environment: The external setting with which the agent interacts.
Action: A move the agent can make.
Reward: Feedback that evaluates the consequence of actions.
Machine Learning Applications
Machine learning has been integrated into various domains, transforming processes and enabling predictive accuracy beyond traditional techniques.
Healthcare and Diagnostics
In medical technology, machine learning enhances diagnostic capabilities by analyzing visual and numerical data. For example, algorithms interpret radiographic images to detect irregular tissue formations or track disease progression. Patient history data is also used to predict the likelihood of specific medical events.
Financial Modeling and Risk Analysis
Financial institutions rely on ML systems for asset management, fraud detection, and loan risk evaluation. These models process transaction patterns, identify anomalies, and flag behaviors that deviate from established norms.
Retail Optimization and Personalization
Retail systems incorporate machine learning to personalize customer experiences. User interaction data is processed to determine product recommendations, optimize marketing strategies, and fine-tune inventory forecasts.
Transportation and Autonomous Navigation
Autonomous driving platforms utilize ML to evaluate sensor inputs and perform contextual decision-making. Machine learning enables real-time route adjustments and object recognition, contributing to vehicle safety and navigational accuracy.
Natural Language and Communication Systems
In text processing and language interpretation, ML models support conversational agents, speech recognition platforms, and automatic translation tools. These systems decode syntax, infer sentiment, and generate human-like responses.
Advantages of Machine Learning
The growing presence of machine learning across industries stems from its diverse capabilities and advantages.
Task Automation
Repetitive operations across logistics, data entry, and diagnostics are increasingly automated through ML models. These systems execute tasks continuously without fatigue, leading to operational efficiency.
Predictive Accuracy
By identifying relationships in datasets, machine learning systems can anticipate outcomes with considerable precision. This predictive power is useful in areas like demand planning and equipment maintenance forecasting.
Customization at Scale
User data enables systems to tailor experiences individually. This personalization enhances user engagement across digital platforms, from media streaming services to e-commerce stores.
Rapid Adaptation to New Data
Once trained, ML models can update their parameters in response to fresh information, maintaining relevance without requiring reprogramming from developers.
Limitations and Concerns in Machine Learning
Despite its benefits, machine learning faces practical and ethical hurdles.
Data Sensitivity and Privacy
Access to user behavior, health, or financial data presents privacy risks. Machine learning systems must be governed by frameworks that ensure ethical data handling and secure data transmission.
Interpretability Challenges
Some machine learning methods, particularly deep learning, produce models whose decision-making logic is opaque. This lack of transparency can hinder user trust and accountability.
Algorithmic Bias
Models trained on unbalanced or skewed data may internalize and perpetuate existing societal biases. Careful dataset curation and model auditing are necessary to address this issue.
Computational Resource Demands
Training complex models, especially those involving large neural networks, requires substantial computational infrastructure. This can be a limiting factor for smaller organizations.
Generalization Limits
While models may perform well on training datasets, they can struggle to generalize when confronted with new or differently structured data. Techniques like cross-validation are used to assess and mitigate this risk.
Evolving Techniques and Future Directions
Ongoing research in machine learning aims to address current limitations and expand model capabilities.
Meta-Learning
Meta-learning refers to systems that improve their ability to learn new tasks with minimal data. These models are designed to adapt quickly, a key advantage in dynamic environments.
Federated Learning
Federated learning allows multiple devices or entities to collaborate on model training without exchanging raw data. This decentralized method supports data privacy while leveraging shared learning objectives.
Explainable AI (XAI)
XAI focuses on making model decisions understandable to humans. Techniques include saliency maps, feature importance scoring, and decision path tracing.
Self-Supervised Learning
Self-supervised learning reduces the need for manually labeled data. Models use data’s internal structure to create pseudo-labels, enabling training at scale.
Hybrid Systems
The integration of symbolic reasoning with statistical learning aims to combine the interpretability of logic-based systems with the adaptability of data-driven models. This hybrid approach is being explored to enhance reasoning capabilities in ML systems.