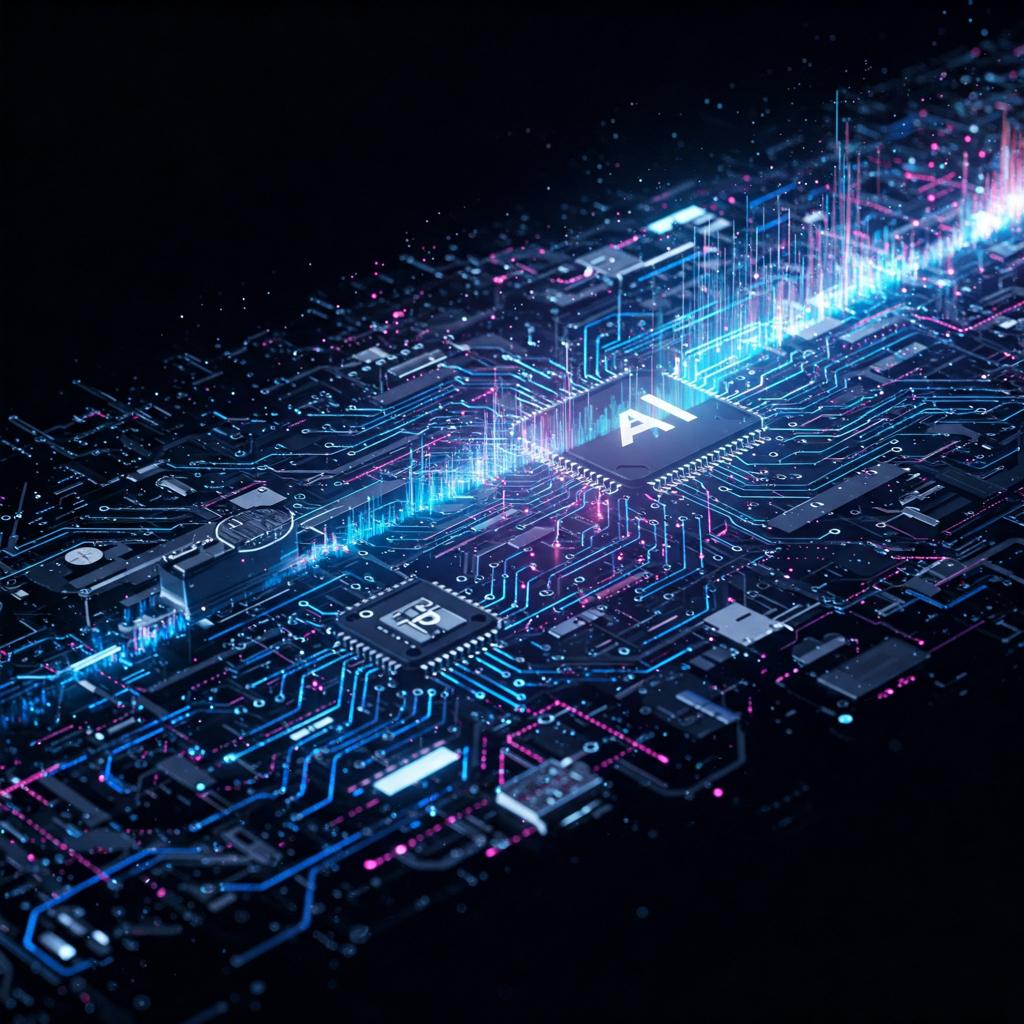
Reactive AI: How It Shapes Modern Technology
Reactive AI refers to a category of artificial intelligence systems that are designed to respond to specific stimuli or inputs in real-time without storing past experiences. Unlike more advanced AI systems, Reactive AI does not retain memory or learn from past interactions. Instead, it focuses on solving immediate problems based on predefined rules or patterns. These AI systems are highly specialized in performing specific tasks but lack the ability to adapt or generalize beyond their programmed functions.
Characteristics of Reactive AI
Reactive AI is characterized by its simplicity and task-focused design. Below are some of the defining features of Reactive AI that set it apart from more complex forms of artificial intelligence:
No Memory or Learning
One of the key features of Reactive AI is its lack of memory. Unlike other forms of AI that improve through learning and adapting over time, Reactive AI systems operate based on the input received at the moment. They do not retain information or experiences from past interactions. This characteristic makes Reactive AI less flexible compared to systems that rely on data retention to optimize performance.
Task-Specific
Reactive AI systems are designed for specific tasks. They excel in performing predefined functions and solving problems that fall within a narrowly defined scope. However, they are not capable of generalizing knowledge across tasks or handling new, unanticipated situations. This makes Reactive AI suitable for environments where tasks are repetitive and predictable.
Real-Time Response
Reactive AI systems are optimized for real-time operations. These systems process inputs and generate outputs almost instantaneously. The response time of Reactive AI is typically very low, allowing it to respond quickly to changing inputs or conditions without delay.
Rule-Based Actions
The behavior of Reactive AI is governed by a set of predefined rules or algorithms. These rules determine how the system will react to different types of stimuli. Because Reactive AI does not incorporate learning from past events, its actions are entirely dependent on the specific rules it has been programmed to follow.
Examples of Reactive AI
Although Reactive AI is not as advanced as other forms of artificial intelligence, it is highly effective for many practical applications. Below are several examples of Reactive AI systems in use today:
1. Chess-Playing AI
Early examples of chess-playing AI, such as IBM’s Deep Blue, are prime instances of Reactive AI. These systems utilize fixed algorithms to evaluate the current state of a chessboard and select a move based on predefined rules. Deep Blue, for example, did not adapt or learn from previous matches but simply followed a set of logical patterns to assess the game and choose the best possible moves at any given moment.
2. Autonomous Robots in Manufacturing
In industrial settings, Reactive AI is used in robots that perform repetitive tasks, such as assembly, welding, and packaging. These robots rely on real-time sensor data to react to their environment and follow programmed instructions. For instance, a robot in an assembly line may perform the same series of tasks repeatedly, without any need to learn or improve its actions over time. These robots are highly effective in environments where tasks are fixed and predictable.
3. Customer Service Chatbots
Customer service chatbots that handle basic inquiries and customer support tasks are another common application of Reactive AI. These chatbots typically respond to customer questions based on keywords or predetermined responses. They do not learn from past interactions, meaning that each query is treated as an isolated event. As a result, the chatbot cannot adapt to different conversational patterns or adjust responses based on previous interactions with the same user.
4. Autonomous Vehicles (Basic Models)
Some basic autonomous vehicles, particularly those operating in controlled environments such as warehouses or factories, utilize Reactive AI. These vehicles are equipped with sensors that allow them to navigate simple paths while responding to obstacles in real time. However, these basic models do not adapt or learn from previous experiences, meaning they are limited to operating in structured, predictable environments where little change occurs.
5. Image Recognition Systems
Certain image recognition technologies, such as those found in security cameras, are powered by Reactive AI. These systems detect specific visual cues, such as motion, and trigger a response, such as an alarm, without retaining any data about previous events. For example, a camera might be programmed to recognize movement in a restricted area and immediately alert security personnel, but it will not remember past events once they have been processed.
Benefits of Reactive AI
While Reactive AI is limited in its scope, it still provides several advantages in specific applications. Below are some of the key benefits of Reactive AI:
Simplicity
One of the primary advantages of Reactive AI is its simplicity. These systems are relatively easy to design, program, and implement. Since Reactive AI does not involve complex algorithms or learning processes, it can be a cost-effective solution for tasks that require straightforward decision-making.
Efficiency
Reactive AI systems are designed for immediate responses, making them highly efficient in real-time environments. Because they operate without the need for long-term processing or memory, they require minimal computational resources, making them ideal for applications where efficiency is crucial.
Consistency
Due to the fixed rules that govern Reactive AI, these systems are highly consistent in their performance. They will always respond to specific inputs in the same way, making them reliable for applications where uniformity and predictability are essential.
Reliability
Another benefit of Reactive AI is its reliability. Since these systems do not rely on learning from past experiences, they are less likely to encounter issues such as overfitting, data bias, or errors related to the misinterpretation of historical data. This makes Reactive AI particularly useful in high-stakes environments where consistency and accuracy are essential.
Limitations of Reactive AI
Despite its advantages, Reactive AI has several limitations that restrict its use in more complex applications:
No Adaptability
One of the most significant limitations of Reactive AI is its inability to adapt to new situations. Since these systems do not learn from past experiences or adjust based on changing conditions, they are suited only for environments where tasks are predictable and static.
Lack of Memory
Reactive AI systems do not have memory, meaning they cannot make decisions based on prior knowledge or historical data. This limits their ability to solve complex problems that require an understanding of previous interactions or events.
Task-Specific
Reactive AI is highly specialized in performing specific tasks. While this makes it highly effective for certain applications, it also means that Reactive AI cannot be easily repurposed for new or dynamic tasks. For instance, a chatbot designed to answer questions about one product cannot seamlessly transition to answering queries about an entirely different product without significant reprogramming.
No Creativity
Reactive AI systems do not have the ability to think creatively. Their responses are strictly determined by the rules and algorithms they follow, meaning they cannot innovate or generate new ideas. This makes Reactive AI unsuitable for tasks that require out-of-the-box thinking or the ability to address novel situations.
The Role of Reactive AI in the Future
Despite its limitations, Reactive AI is likely to continue playing an important role in the future of various industries. As more advanced AI systems develop, Reactive AI will still be highly useful for specific applications that require real-time responses and consistency.
In particular, Reactive AI will remain essential in fields such as manufacturing, where repetitive tasks are common, and customer service, where efficiency is key. Additionally, the simplicity and reliability of Reactive AI make it an excellent tool for specialized systems that do not require the ability to learn or adapt.
While Reactive AI is unlikely to evolve into more advanced forms of AI, it may integrate into more complex systems that include machine learning and memory. In these hybrid systems, Reactive AI will serve as a foundational component, offering real-time responses while more advanced algorithms handle learning and adaptation.
As the field of artificial intelligence continues to evolve, Reactive AI may be combined with other types of AI to create systems that offer both immediate responses and the ability to learn from past experiences. However, for the foreseeable future, Reactive AI will remain a key technology in areas where speed, efficiency, and consistency are paramount.