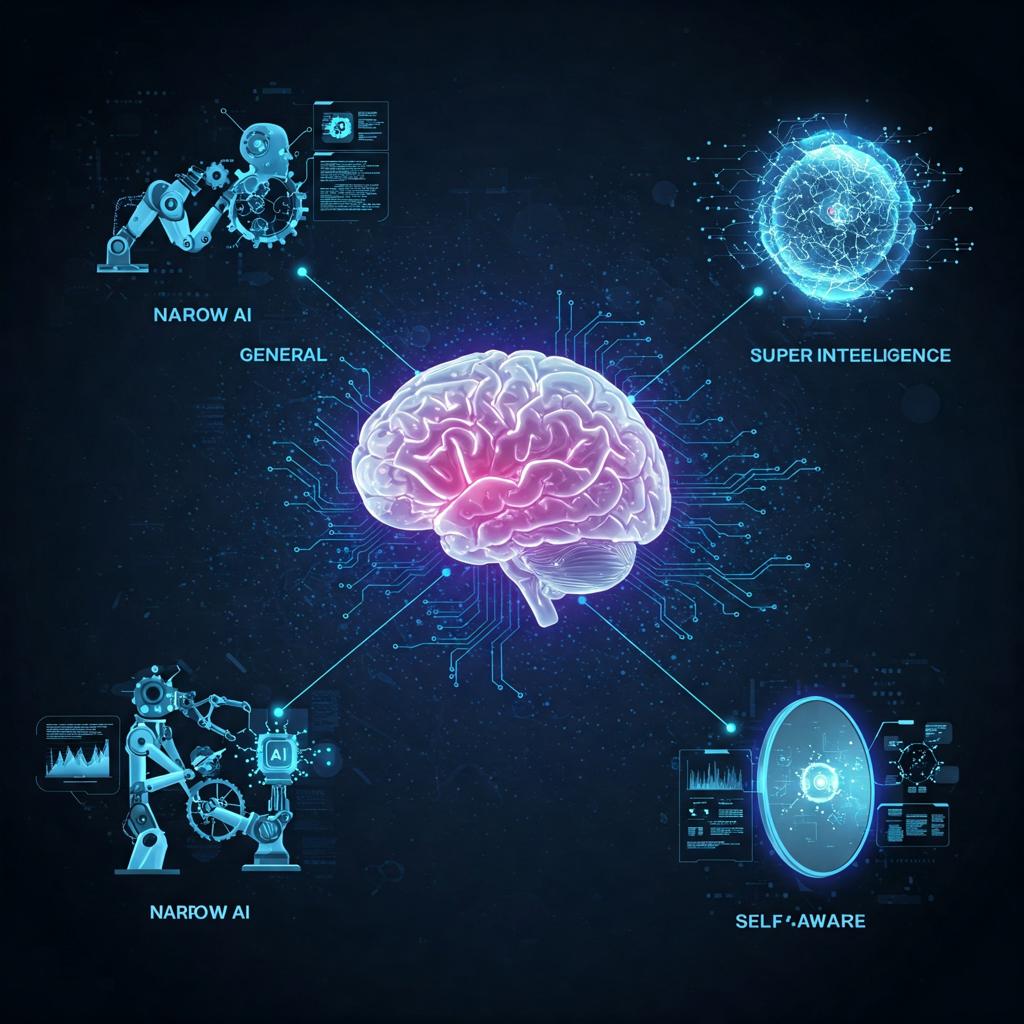
Understanding the Different Types of AI Explained
There are different types of artificial intelligence (AI), and they can be classified in various ways depending on their capabilities, learning methods, and areas of application.
1. Weak AI (Narrow AI)
This is artificial intelligence that is designed to perform a specific task. It is very common today and includes applications such as search engines, recommendation systems, chatbots, self-driving cars, and automated medical diagnostic systems.
- Examples: Siri, Alexa, facial recognition systems, machine translation software, ChatGPT.
Type of Weak AI | Examples | Main Function |
---|---|---|
Speech and language recognition | Siri, Alexa, Google Assistant | Interpret and respond to voice commands |
Facial and image recognition | Face ID, smart surveillance systems | Identify faces, objects, and environments |
Chatbots and virtual assistants | Bank chatbots, website helpdesk bots | Automate conversations and provide support |
Search engines and recommendation systems | Google Search, Netflix, Amazon | Suggest personalized content or products |
Basic autonomous driving | Tesla Autopilot (Level 2-3) | Assist with driving, parking, and automatic braking |
AI in video games | NPCs in FIFA, GTA, The Last of Us | Simulate realistic and adaptive in-game behaviors |
AI-assisted medical diagnosis | IBM Watson Health, Aidoc | Support medical diagnosis and image analysis |
Fraud detection | Banking and financial fraud detection AI | Analyze and block suspicious transactions |
Automated financial trading | Crypto bots, stock market algorithms | Make fast investment decisions based on data |
Machine translation | Google Translate, DeepL | Provide real-time translation with increasing accuracy |
2. Strong AI (General AI)
This is theoretical artificial intelligence that possesses human-like cognitive abilities, capable of understanding, learning, and applying knowledge in different contexts. There are no concrete examples of strong AI yet; it is a future direction in AI research.
- Example: A system that could, in theory, perform any human cognitive task, such as solving complex problems, understanding emotions, and making decisions independently.
Type of Strong AI (General AI) | Description |
---|---|
Cognitive Architecture-based AI | Models inspired by human cognition, such as SOAR or ACT-R, simulating general intelligence mechanisms. |
Whole Brain Emulation (WBE) | Simulates the entire structure and function of the human brain digitally. |
Integrated Learning Systems | Combines multiple learning paradigms to adapt across diverse tasks like a human. |
Embodied AI | AI with a physical or simulated body that learns through interaction with the environment. |
Self-improving AI | AI that autonomously enhances its own algorithms and knowledge base over time. |
Artificial Consciousness | Theoretical AI capable of self-awareness, emotions, and understanding its own existence. |
3. Superintelligence
This refers to a form of artificial intelligence that surpasses human intellectual capabilities in virtually all areas, including creativity, complex problem solving, and the ability to learn and adapt. This type of AI is purely theoretical and remains a future vision in AI discussions.
- Example: An AI that is significantly more intelligent than any human being in every field, including areas such as science, medicine, and philosophy.
Type of Superintelligence | Description |
---|---|
Speed Superintelligence | Processes information and makes decisions far faster than the human brain. |
Collective Superintelligence | Emerges from networks of AI systems working together with shared intelligence. |
Quality Superintelligence | Possesses cognitive abilities far superior to the best human minds in every field. |
Artificial Superintelligence (ASI) | Fully autonomous intelligence surpassing human reasoning, creativity, and problem-solving. |
Recursive Self-improving AI | Continuously improves its own design, leading to rapid and exponential intelligence growth. |
Strategic Superintelligence | Excels in long-term planning, manipulation, and achieving complex goals with high efficiency. |
4. Reactive AI
This type of AI is designed to respond to specific inputs without storing past experiences. These artificial intelligences do not have a ‘memory’ and act only on the basis of current information.
- Example: Games such as IBM's Deep Blue, which can play chess but does not retain memory of previous games.
Type of Reactive AI | Description |
---|---|
Simple Reactive Machines | Respond to current inputs with predefined rules, without memory or learning. |
Rule-Based Reactive Systems | Operate based on a set of fixed rules triggered by specific stimuli. |
Sensor-Based Reactive AI | Uses real-time sensor data to make immediate decisions, common in robotics. |
Finite State Machines | Transition between states based solely on current input conditions. |
Game-Playing Reactive AI | Makes decisions in real-time based on the current game state (e.g., Deep Blue). |
5. Limited Memory AI
This artificial intelligence has the ability to store some information to make better decisions over time. For example, self-driving cars use limited memory AI to remember previous situations and improve navigation.
- Example: Autonomous vehicles that learn from past experiences to improve their decision-making abilities.
Type of Limited Memory AI | Description |
---|---|
Supervised Learning AI | Learns from historical data labeled by humans to make predictions. |
Unsupervised Learning AI | Analyzes patterns and structures in unlabeled data for clustering or association. |
Reinforcement Learning AI | Learns through trial and error using rewards and penalties. |
Semi-Supervised Learning AI | Combines small amounts of labeled data with large amounts of unlabeled data. |
Time-Series Predictive AI | Uses historical time-based data to forecast future outcomes. |
Self-Driving Car Systems | Apply real-time data and limited past experiences to make driving decisions. |
6. Theory of Mind AI
This type of AI refers to the ability to understand and simulate the mental states of humans, such as thoughts, emotions, intentions, and perceptions. It is a future direction of artificial intelligence and is based on the idea that AI can interact more naturally and empathetically with humans.
- Example: A robot or assistant that understands how a person feels and adapts its behaviour accordingly.
Type of Theory of Mind AI | Description |
---|---|
Emotion Recognition AI | Identifies and responds to human emotions through voice, facial expressions, or behavior. |
Belief Modeling AI | Simulates and interprets human beliefs, intentions, and desires. |
Cognitive Modeling AI | Mimics human thought processes to predict behavior in complex situations. |
Social Interaction AI | Engages in dynamic, context-aware conversations considering social cues. |
Perspective-Taking AI | Understands and reacts based on another entity’s point of view or context. |
Adaptive Human-Aware Systems | Adjusts behavior in response to users’ mental states and social signals. |
7. Self-aware AI
This is an advanced form of AI that, in addition to understanding and simulating emotions and thoughts, is aware of itself. This type of artificial intelligence is still theoretical and does not currently exist.
- Example: A system that is aware of its own existence, its limitations, and its internal state.
Type of Self-aware AI | Description |
---|---|
Proto-Self AI | Possesses basic awareness of internal states, such as performance or energy levels. |
Self-Monitoring AI | Tracks its own operations and outcomes to adjust actions autonomously. |
Meta-Cognitive AI | Understands and evaluates its own thought processes and decision-making. |
Reflective AI | Analyzes past actions and experiences to improve future behavior and reasoning. |
Emotionally Self-Aware AI | Recognizes its own simulated emotional states and adjusts interactions accordingly. |
Fully Conscious AI | Hypothetical AI with complete self-awareness, emotions, and subjective experience. |
8. Machine learning (ML)
This is one of the most common technologies in use today. Machine learning algorithms allow systems to learn from data and improve autonomously over time without being explicitly programmed for each task. It is the basis of many modern artificial intelligence applications.
- Example: Classification algorithms to identify objects in images, recommendation systems such as those used by Netflix and Amazon.
Type of Machine Learning (ML) AI | Description |
---|---|
Supervised Learning | Trains on labeled data to make predictions or classify new data. |
Unsupervised Learning | Finds patterns and structures in unlabeled data without predefined outputs. |
Semi-Supervised Learning | Combines small amounts of labeled data with large amounts of unlabeled data. |
Reinforcement Learning | Learns through trial and error, receiving rewards or penalties for actions. |
Deep Learning | Uses neural networks with many layers to model complex patterns and representations. |
Transfer Learning | Applies knowledge from one domain to solve problems in a different but related domain. |
Online Learning | Continuously learns and updates as new data becomes available. |
Active Learning | Selects the most informative data to be labeled and added to the training set. |
Ensemble Learning | Combines multiple models to improve prediction accuracy. |
9. Deep Learning
A subset of machine learning that uses complex artificial neural networks to model deeper and more complex data structures. It is used for tasks such as speech recognition, computer vision and machine translation.
- Example: Facial recognition systems, deep neural network-based machine translators, autonomous vehicles.
Type of Deep Learning | Description |
---|---|
Convolutional Neural Networks (CNNs) | Primarily used for image recognition and processing visual data. |
Recurrent Neural Networks (RNNs) | Designed for sequential data, such as time series or natural language processing. |
Long Short-Term Memory (LSTM) Networks | A type of RNN that overcomes issues with long-term dependencies in sequential data. |
Generative Adversarial Networks (GANs) | Consists of two neural networks (generator and discriminator) to create realistic data. |
Autoencoders | Used for data compression and noise reduction by learning efficient representations. |
Deep Belief Networks (DBNs) | A type of generative model used for unsupervised learning and pretraining deep networks. |
Transformer Networks | Focused on sequential data, particularly in natural language processing, with attention mechanisms. |
Capsule Networks | Aims to improve CNNs by using capsules to recognize objects in various orientations. |
Neural Turing Machines (NTMs) | A neural network combined with an external memory to perform tasks like algorithm execution. |
Deep Reinforcement Learning | Combines deep learning with reinforcement learning to enable decision-making from high-dimensional inputs. |
10. Logic-based AI (Expert Systems)
This is a form of AI that uses a knowledge base and predefined rules to solve specific problems. These systems do not ‘learn’ autonomously, but operate on logic and rules provided by humans.
- Example: Decision support systems, such as those used in medicine to diagnose diseases.
Type of Logic-based AI (Expert Systems) | Description |
---|---|
Rule-Based Expert Systems | Uses predefined rules and logic to make decisions based on input data. |
Knowledge-Based Systems | Relies on a large knowledge base to derive conclusions and solve problems. |
Fuzzy Logic Systems | Handles uncertainty and imprecision in decision-making with "degrees of truth." |
Decision Support Systems | Aids decision-making by analyzing complex data using expert knowledge and logic. |
Inference Engines | A component of expert systems that applies logical rules to the knowledge base to derive new information. |
Case-Based Reasoning | Solves problems by retrieving similar past cases and applying the same solutions. |
Heuristic-based Systems | Uses heuristics or rules of thumb to make educated guesses for complex problems. |
Constraint-Based Systems | Solves problems by considering constraints and selecting solutions that satisfy them. |
The future of AI
The future of AI lies not only in automation but in collaboration—where intelligent systems become creative partners, ethical advisors, and adaptive learners. As AI evolves beyond task execution, it may grow into a mirror of human curiosity, shaping a world where machines understand nuance, context, and perhaps even empathy.