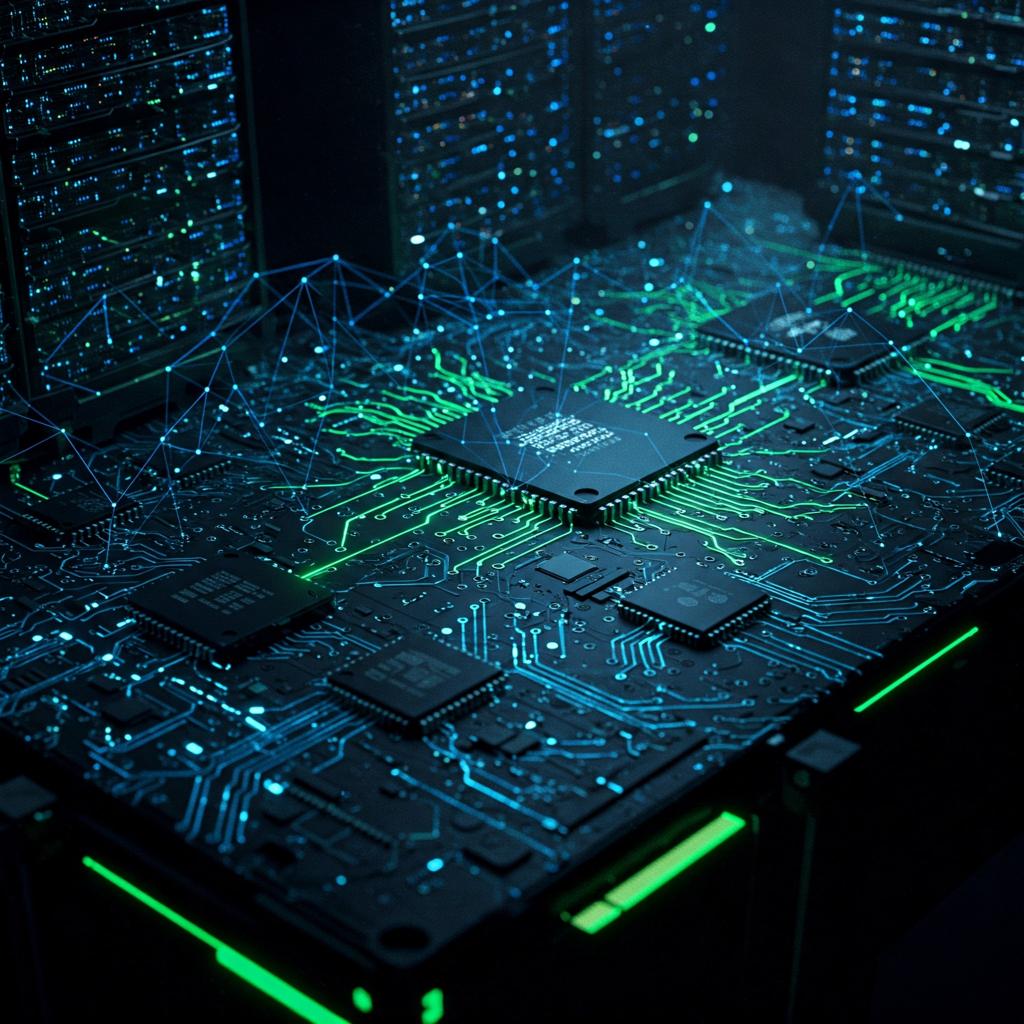
What types of AI are ChatGPT and its Competitors
ChatGPT, a cutting-edge AI language model developed by OpenAI, has taken the world by storm. Built on the foundation of large-scale language models (LLMs), it has brought conversational AI to new heights, offering capabilities such as generating human-like text, answering questions, and assisting with complex tasks. This article provides a detailed breakdown of what ChatGPT is, how it works, and compares it with other similar AI models. Additionally, we will discuss the hardware and infrastructure necessary to run a model like ChatGPT.
What is ChatGPT?
At its core, ChatGPT is a Large Language Model (LLM). These models are designed to process and generate human-like text by predicting the next word or token in a sequence, based on the context of the preceding text. ChatGPT, specifically, uses a version of the GPT (Generative Pre-trained Transformer) architecture, which employs a decoder-only Transformer design. This structure makes it particularly adept at generating coherent and contextually relevant outputs.
Unlike traditional models designed for tasks such as classification or encoding, a decoder-only Transformer is tailored for autogenerative tasks. That is, it generates output one token at a time, iteratively predicting the next word based on the words that came before it. This allows the model to create fluid, coherent sentences and paragraphs, making it ideal for applications like chatbots, content generation, and more.
How ChatGPT Works: Key Features
Autoregressive Model
ChatGPT is an autoregressive model, which means it generates one token at a time, predicting the next based on the sequence of tokens it has seen so far. This is the hallmark of many LLMs, where the model’s predictions are informed entirely by the context provided by the previous tokens in the conversation or text.
In simpler terms, ChatGPT doesn't rely on "predefined" responses. Instead, it dynamically constructs its output based on input data, which makes it highly versatile for generating text in a wide range of contexts.
Transformer Architecture
The Transformer architecture is at the heart of ChatGPT. Unlike earlier models, such as RNNs and LSTMs, which process data sequentially, the Transformer uses an attention mechanism to handle dependencies between words regardless of their distance in the input text. However, ChatGPT specifically uses a decoder-only Transformer architecture. This means that, while Transformers can be used for both encoding and decoding tasks (as in machine translation), a decoder-only model is solely focused on generating text from the given context.
The decoder-only design enables the model to focus entirely on output generation, predicting the next word or token based on previously seen data. This makes it highly efficient for tasks requiring text generation, such as dialogue systems, creative writing, and question-answering.
Pretraining and Fine-Tuning
ChatGPT undergoes an extensive pretraining phase, during which it is exposed to vast amounts of text data in an unsupervised manner. This pretraining allows the model to learn the underlying structure of human language, understanding grammar, syntax, and even some factual knowledge embedded in the data it processes.
After pretraining, ChatGPT is fine-tuned using a method called Reinforcement Learning from Human Feedback (RLHF). This step is crucial, as it refines the model's behavior based on human feedback, ensuring that the model provides more accurate and contextually appropriate responses. Through RLHF, the model is better able to handle ambiguous questions, make ethical judgments, and provide more coherent responses to complex queries.
Zero-Shot and Few-Shot Learning
One of the standout features of ChatGPT is its ability to generalize across tasks with minimal task-specific training. This is known as zero-shot or few-shot learning. In a zero-shot scenario, the model can handle a new task without requiring any explicit retraining or fine-tuning. It simply relies on its pre-existing knowledge from its pretraining and fine-tuning phases.
In few-shot learning, the model can perform tasks effectively with only a few examples provided during the interaction. This is why ChatGPT is so versatile, as it can quickly adapt to different conversational contexts, from casual chats to more technical or specialized topics.
Token-Based Processing
ChatGPT operates on a token-based system. When you input a sentence, the model first breaks it down into smaller pieces known as tokens, which are usually subwords or words. These tokens are then processed by the model’s neural network, which predicts the next token based on the previous ones. This is done through an iterative process until the full output is generated.
Tokens are the basic units of input and output in a model like ChatGPT. This approach allows for efficient processing, as the model doesn't need to store entire sentences in memory but instead works with smaller, more manageable pieces of information.
Wide Context Window
ChatGPT is designed with a wide context window, meaning it can process large chunks of text at once. For example, depending on the version of the model, ChatGPT can handle input sequences that span tens of thousands of tokens. This allows the model to keep track of long conversations, generate long-form content, and understand the broader context of an interaction, which is essential for maintaining coherence and relevance in extended dialogues.
Stateless by Default
By design, ChatGPT is stateless. This means that once a session ends, the model does not retain any memory of previous conversations. Each new interaction begins with a clean slate, ensuring privacy and reducing the risk of data leakage. However, this also means that ChatGPT doesn't "remember" past interactions, making it less personalized but more secure.
For some users, memory features are being tested, allowing the model to recall past conversations within the same session or across multiple interactions. These features are still being refined and are not yet universally available.
Hardware Requirements for Running a Model Like ChatGPT
Running a model as sophisticated as ChatGPT requires significant computational resources. The hardware infrastructure needed can be broken down into the following components:
GPUs
The primary hardware used for training and inference of models like ChatGPT is Graphics Processing Units (GPUs). These specialized processors are designed to handle the massive parallel computations required for deep learning models. In particular, high-end GPUs like the NVIDIA A100 or H100 are used for training large models. These GPUs provide the necessary processing power to train the model on billions of parameters.
For inference (the process of generating responses to queries), GPUs are also crucial to ensure that responses are generated quickly and efficiently, especially in a production setting where users expect low-latency interactions.
High-Bandwidth Networking
Because models like ChatGPT often operate on a distributed infrastructure, high-bandwidth networking is critical. The GPUs used for training and inference are typically connected via high-speed interconnects like NVLink or InfiniBand, which ensure that data can be transferred quickly between processors, enabling faster training times and smoother inference.
Data Storage
Training large-scale models requires massive amounts of data. To store this data efficiently, high-performance storage systems with fast read and write speeds are necessary. These storage solutions must be able to handle petabytes of data without bottlenecking the training process.
Clusters of Servers
To handle the computational demands of training and inference, AI models like ChatGPT are typically run on clusters of interconnected servers. These clusters contain numerous GPUs and CPUs, all working in parallel to execute the computations required by the model. Large-scale AI research and companies often utilize cloud infrastructure or their own on-premises data centers to run these clusters.
To give you an example
To ensure that an AI like ChatGPT functions effectively, the underlying system must be robust and capable of handling the immense computational demands. One such system is the DGX A100, a high-performance machine developed by NVIDIA, specifically designed for deep learning and AI workloads. These systems are equipped with powerful NVIDIA A100 Tensor Core GPUs, which provide the massive parallel processing power required to train and run large-scale AI models efficiently. However, acquiring a system like the DGX A100 is not inexpensive; it involves significant investment, as these systems can cost hundreds of thousands of dollars depending on configuration and scale.
In addition to the hardware, an AI like ChatGPT requires a comprehensive infrastructure to function seamlessly. This includes both a front end and a back end. The front end handles the user interface and the interactions with the AI, providing a smooth and responsive experience for users. It is responsible for processing inputs, displaying results, and ensuring that the AI's output is accessible in a user-friendly manner.
On the other hand, the back end consists of the servers, databases, and network infrastructure that support the AI’s processing and data storage. It manages tasks such as running the AI model, handling requests, ensuring scalability, and storing massive amounts of training data. The back end also includes powerful cloud computing platforms or data centers where all of the heavy computations and storage occur. These systems work in tandem with the front end to ensure that users receive fast, accurate, and reliable responses from the AI.
Altogether, this complex system of hardware and software is critical for the successful deployment and operation of AI models like ChatGPT. The high costs and intricate setup reflect the cutting-edge technology and infrastructure required to bring such advanced artificial intelligence to life.
While a powerful system like the DGX A100 is essential for running an AI model like ChatGPT, it is not sufficient to handle requests from multiple users simultaneously, especially at a large scale. To support thousands or even millions of concurrent users, the system must be part of a much larger infrastructure that includes clustering and load balancing.
When a single DGX A100 is tasked with running an AI model, it can handle requests from only a limited number of users due to hardware limitations like processing power, memory, and GPU capacity. However, for AI systems like ChatGPT to function in a real-world environment, where numerous users are interacting with the AI at the same time, additional components are necessary.
Clustered systems involve multiple powerful machines (often multiple DGX A100 units or similar high-performance servers) working together to process tasks in parallel. These machines are connected within a high-performance computing cluster, distributing the computational load across several nodes. This ensures that the system can handle more substantial traffic, providing the necessary resources to handle complex computations and serve multiple users simultaneously.
To effectively manage the workload across these multiple machines, load balancing becomes a crucial component of the architecture. Load balancing distributes incoming user requests evenly across the available servers, ensuring that no single machine becomes overwhelmed. This method improves efficiency and reduces latency by ensuring that computational resources are used optimally. The system automatically adjusts to changes in demand, scaling up or down as needed, making it highly flexible and responsive.
Moreover, scaling such systems typically requires cloud infrastructure or data center services that support horizontal scaling. Horizontal scaling allows the infrastructure to expand by adding more servers or computational resources rather than upgrading individual machines. This enables a more efficient and cost-effective approach to handling large-scale user interactions, as additional servers can be added to the cluster when demand increases, and removed when demand decreases.
In summary, while the DGX A100 is a powerful tool for running AI models, it is only one piece of the puzzle. To support multiple users and ensure smooth, responsive service, distributed systems, clustering, and load balancing are essential. These components work together to provide the scalability and reliability needed for large-scale AI applications like ChatGPT.
Competitors to ChatGPT
While ChatGPT is a leading AI model in the realm of conversational agents, it faces competition from other state-of-the-art models developed by various companies and research organizations. Some notable alternatives include:
Google’s Bard: Built on Google’s LaMDA (Language Model for Dialogue Applications), Bard offers conversational capabilities similar to ChatGPT, with a focus on real-time search results.
Anthropic’s Claude: A language model that emphasizes safety and alignment in AI, Claude aims to make AI more interpretable and less prone to harmful outputs.
Meta’s LLaMA: Meta’s open-weight large language model is designed for a range of tasks and is built to be more efficient in handling resource-intensive applications.
Final Thoughts
ChatGPT and its competitors represent the cutting edge of AI technology, providing humans with powerful tools for communication, creativity, and problem-solving. While the underlying architectures of these models—like autoregressive generation, Transformer design, and token-based processing—are relatively similar, each model has its own approach to fine-tuning, training, and deployment. With the right hardware infrastructure and continuous improvements in AI research, these models will continue to evolve, driving innovation in both AI and human-machine interaction.